ITEMS Portal
Digital Module 02: Scale Reliability in Structural Equation Modeling
4 (7 votes)
Recorded On: 06/14/2019
-
Register
- Learner - Free!
In this digital ITEMS module, we frame the topic of scale reliability within a confirmatory factor analysis and structural equation modeling (SEM) context and address some of the limitations of Cronbach’s α. This modeling approach has two major advantages: (1) it allows researchers to make explicit the relation between their items and the latent variables representing the constructs those items intend to measure, and (2) it facilitates a more principled and formal practice of scale reliability evaluation. Specifically, we begin the module by discussing key conceptual and statistical foundations of the classical test theory (CTT) model and then framing it within an SEM context; we do so first with a single item and then expand this approach to a multi-item scale. This allows us to set the stage for presenting different measurement structures that might underlie a scale and, more importantly, for assessing and comparing those structures formally within the SEM context. We then make explicit the connection between measurement model parameters and different measures of reliability, emphasizing the challenges and benefits of key measures while ultimately endorsing the flexible McDonald’s ω over Cronbach’s α. We then demonstrate how to estimate key measures in both a commercial software program (Mplus) and three packages within an open-source environment (R). In closing, we make recommendations for practitioners about best practices in reliability estimation based on the ideas presented in the module.
Keywords: congeneric, Cronbach’s alpha, reliability, scale reliability, SEM, structural equation modeling, McDonald’s omega, model fit, parallel, tau-equivalent
Key:





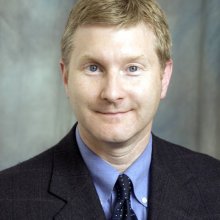
Gregory R. Hancock
Professor
Gregory R. Hancock is Professor, Distinguished Scholar-Teacher, and Director of the Measurement, Statistics and Evaluation program in the Department of Human Development and Quantitative Methodology at the University of Maryland, College Park, and Director of the Center for Integrated Latent Variable Research (CILVR). His research interests include structural equation modeling and latent growth models, and the use of latent variables in (quasi)experimental design. His research has appeared in a wide variety of prestigious peer-reviewed journals. He also co-edited the volumes Structural Equation Modeling: A Second Course (2006; 2013), The Reviewer's Guide to Quantitative Methods in the Social Sciences (2010), and Advances in Longitudinal Methods in the Social and Behavioral Sciences (2012). He has taught dozens of methodological workshops in the United States, Canada, and outside North America and has received the 2011 Jacob Cohen Award for Distinguished Contributions to Teaching and Mentoring by the APA.
Contact Greg via ghancock@umd.edu
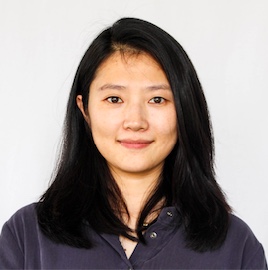
Ji An
Ph.D. Candidate
Ji An is a Ph.D. candidate in the Measurement, Statistics and Evaluation program in the Department of Human Development and Quantitative Methodology at the University of Maryland, College Park. Her research interests include propensity score methods, analysis of survey data with complex sampling designs, structural equation modeling, and multilevel modeling. Before her time at College Park she had earned an M.A. in Teaching and Curriculum from Michigan State University. She has subsequently worked as an instructor for introductory courses in educational statistics and as a graduate assistant for various courses at the undergraduate and graduate level.
Contact Ji via anji.nihao@gmail.com