ITEMS Portal
ACCESS IS FREE!
Quickly create a new user account for the Elevate learning management system (this website) to access any modules.
You do NOT have to be an NCME member to do this!
Bayesian Psychometrics
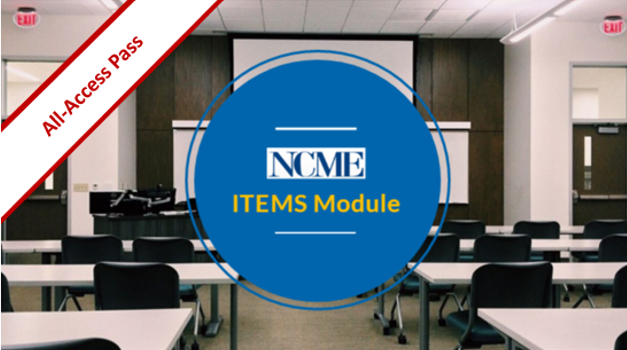
All-access Pass
This provides immediate access to ALL print and digital modules in the portal by "registering" you for each and displaying all modules as a single collection as part of this pass.
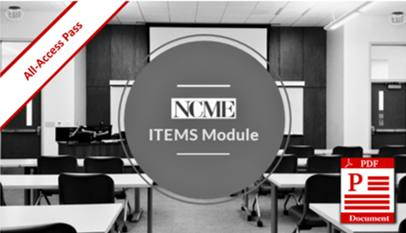
All-access Pass (PRINT ONLY)
This provides access to a ZIP folder with all 45 previously published print modules.
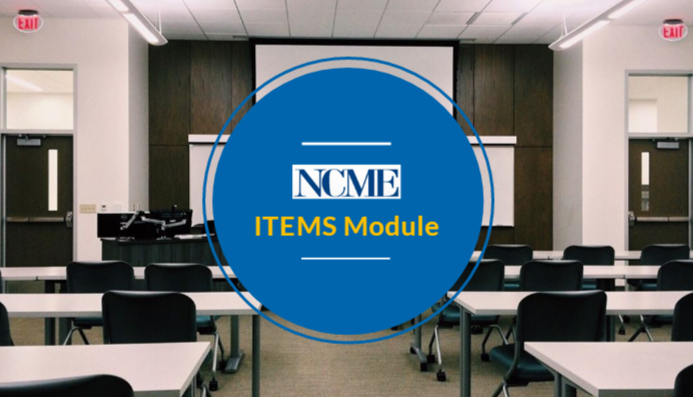
Digital Module 11: Bayesian Psychometrics
In this digital ITEMS module, Dr. Roy Levy discusses how Bayesian inference is a mechanism for reasoning in probability-modeling framework, describes how this plays out in a normal distribution model and unidimensional item response theory (IRT) models, and illustrates these steps using the JAGS software and R.
Keywords: Bayesian psychometrics, Bayes theorem, dichotomous data, item response theory (IRT), JAGS, Markov-chain Monte Carlo (MCMC) estimation, normal distribution, R, unidimensional models
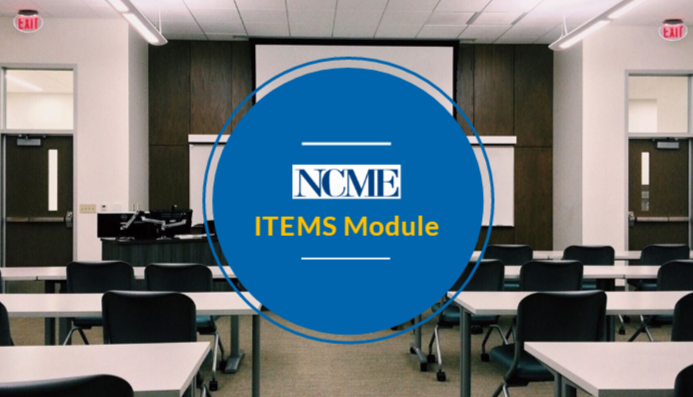
Digital Module 06: Posterior Predictive Model Checking
In this digital ITEMS module, Dr. Allison Ames and Aaron Myers discuss the most common Bayesian approach to model-data fit evaluation, which is called Posterior Predictive Model Checking (PPMC), for simple linear regression and item response theory models.
Keywords: Bayesian inference, simple linear regression, item response theory, IRT, model fit, posterior predictive model checking, PPMC, Bayes theorem, Yen’s Q3, item fit
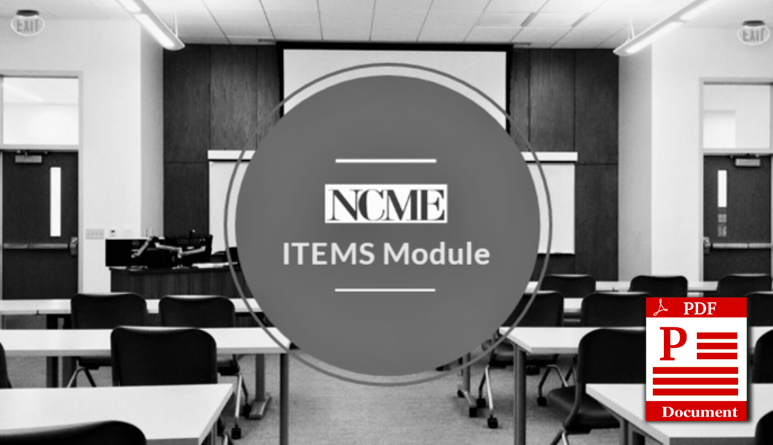
Module 27: Markov Chain Monte Carlo Methods for Item Response Theory Models
In this print module, Dr. Jee-Seon Kim and Dr. Daniel M. Bolt provide an introduction to Markov chain Monte Carlo (MCMC) estimation for item response (IRT) models and illustrate these ideas with a two-parameter logistic (2PL) model in the software program Winbugs.
Keywords: Bayesian estimation, goodness-of-fit, item response theory, IRT, Markov chain Monte Carlo, MCMC, model comparison, two-parameter model, 2PL, Winbugs
Access Date | Quiz Result | Score | Actions |
---|