ITEMS Portal
Digital Module 06: Posterior Predictive Model Checking
3.83 (6 votes)
Recorded On: 01/28/2020
-
Register
- Learner - Free!
In this digital ITEMS module, Dr. Allison Ames and Aaron Myers discuss the most common Bayesian approach to model-data fit evaluation called Posterior Predictive Model Checking (PPMC). Specifically, drawing valid inferences from modern measurement models is contingent upon a good fit of the data to the model and violations of model-data fit have numerous adverse consequences, limiting the usefulness and applicability of the model. As Bayesian estimation is becoming more common, understanding the Bayesian approaches for evaluating model-data fit models is critical. The instructors review the conceptual foundation of Bayesian inference as well as PPMC and walk through the computational steps of PPMC using real-life data examples from simple linear regression and item response theory (IRT) analysis. They provide guidance for how to interpret PPMC results and discuss how to implement PPMC for other model(s) and data. The digital module contains sample data, SAS code, diagnostic quiz questions, data-based activities, curated resources, and a glossary.
Keywords: Bayesian inference, simple linear regression, item response theory, IRT, model fit, posterior predictive model checking, PPMC, Bayes theorem, Yen’s Q3, item fit
Key:





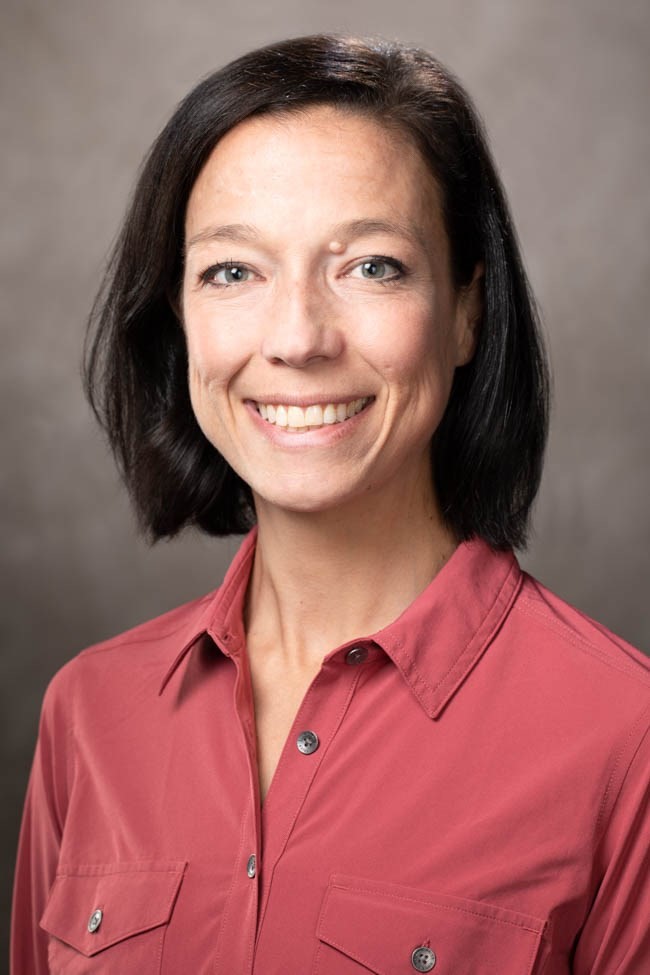
Allison J. Ames
Assistant Professor
Allison is an assistant professor in the Educational Statistics and Research Methods program in the Department of Rehabilitation, Human Resources and Communication Disorders, Research Methodology, and Counseling at the University of Arkansas. There, she teaches courses in educational statistics, including a course on Bayesian inference. Allison received her Ph.D. from the University of North Carolina at Greensboro. Her research interests include Bayesian item response theory, with an emphasis on prior specification; model-data fit; and models for response processes. Her research has been published in prominent peer-reviewed journals. She enjoyed collaborating on this project with a graduate student, senior faculty member, and the Instructional Design Team.
Contact Allison via boykin@uark.edu
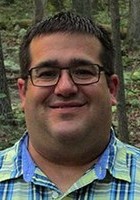
Aaron Myers
Graduate Assistant / Doctoral Student
Aaron is a doctoral student in the Educational Statistics and Research Methods program at the University of Arkansas. His research interests include Bayesian inference, data mining, multidimensional item response theory, and multilevel modeling. Aaron previously received his M.A. in Quantitative Psychology from James Madison University. He currently serves as a graduate assistant where he teaches introductory statistics and works in a statistical consulting lab.
Contact Aaron via ajm045@uark.edu