ITEMS Portal
Digital Module 19: Foundations of IRT Estimation
4.29 (7 votes)
Recorded On: 11/29/2020
-
Register
- Learner - Free!
In this digital ITEMS module, Dr. Zhuoran Wang and Dr. Nathan Thompson introduce the basic item response theory (IRT) item calibration and examinee scoring procedures as well as strategies to improve estimation accuracy. They begin the module with a conceptual review of IRT that includes core advantages of the IRT framework, commonly used IRT models, and essential components such as information and likelihood functions. In the second part of the module, they illustrate the structure and inner workings of calibration and scoring algorithms such as the MMLE/EM algorithm for item parameter calibration and the MLE, EAP, and MAP algorithms for examinee scoring. In part three, they demonstrate the influence of multiple factors on estimation accuracy and provide strategies for maximizing accuracy. In addition to audio-narrated slides, the digital module contains sample R code, quiz questions with diagnostic feedback, curated resources, and a glossary.
Keywords: calibration, EM algorithm, estimation accuracy, item response theory (IRT), maximum likelihood estimation (MLE), maximum a posteriori (MAP), expected a posteriori (EAP), marginal maximum likelihood estimation (MMLE), scoring
Key:





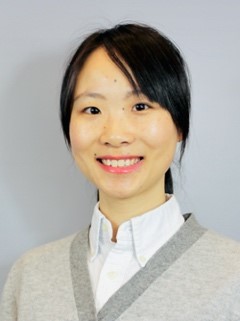
Zhuoran Wang
Prometric
Zhuoran Wang is a psychometrician at Prometric. She provides psychometric expertise for a number of clients, mostly professional associations and state-based licensure exams. Tasks mainly includes classical test theory analysis, item response theory analysis, computerized adaptive testing development, equating, scaling, job analysis, and standard setting. Zhuoran graduated from University of Minnesota with a Ph.D. in Psychometrics/ Quantitative Methods. Her research interests span a wide range of topics in psychometrics including multidimensional item response theory, cognitive diagnostic modeling, differential item functioning, computerized adaptive testing, and multistage testing. When in graduate school, she taught multiple undergraduate level and graduate level statistic and data analysis exercise courses. She also provided R tutorial in two workshops on multidimensional IRT models in IACAT conference 2017 and 2019.
Contact Zhuoran via wang5105@umn.edu
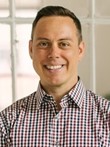
Nathan Thompson
Assessment Systems Corporation (ASC)
Nathan Thompson currently serve as VP for ASC (www.assess.com). His focus is on bringing quality measurement to more organizations, either through software that automates the work, makes it easier to implement sophisticated psychometrics like item response theory, or directly consulting with organizations to ensure that they align with best practices. Nate earned his PhD in Psychometrics from the University of Minnesota, with a focus on computerized adaptive testing. His undergraduate degree was from Luther College with a triple major of Mathematics, Psychology, and Latin. He is primarily interested in the use of artificial intelligence and software automation to augment and replace the work done by psychometricians, which has provided extensive experience in software design and programming. He has published over 100 journal articles and conference presentations. In addition to research, Nate has abundant teaching experiences. He served as an Instructor for the Department of Psychology for two undergraduate statistic courses while in graduate school. When working as adjunct faculty in University of Cincinnati, he taught an online class on measurement and assessment as part of a Master's in Medical Education program.
Contact Nate via nate@assess.com