ITEMS Portal
ACCESS IS FREE!
Quickly create a new user account for the Elevate learning management system (this website) to access any modules.
You do NOT have to be an NCME member to do this!
Item Response Theory
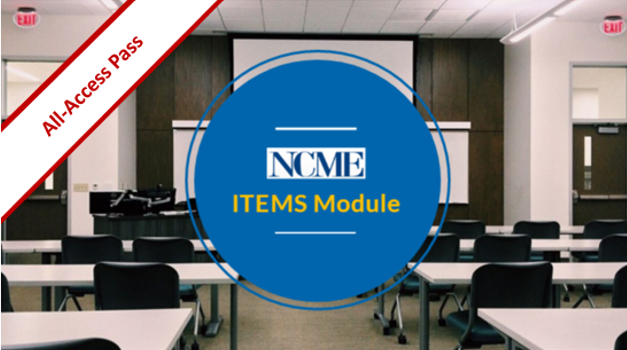
All-access Pass
This provides immediate access to ALL print and digital modules in the portal by "registering" you for each and displaying all modules as a single collection as part of this pass.
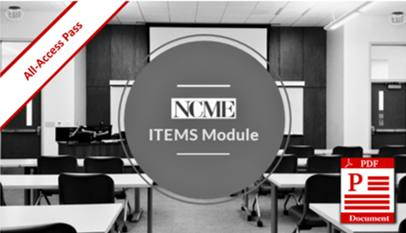
All-access Pass (PRINT ONLY)
This provides access to a ZIP folder with all 45 previously published print modules.
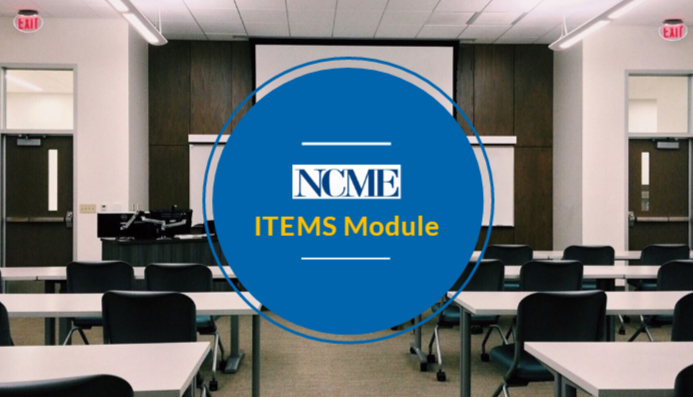
Digital Module 03: Nonparametric Item Response Theory
In this digital ITEMS module Dr. Stefanie Wind introduces the framework of nonparametric item response theory (IRT), in particular Mokken scaling, which can be used to evaluate fundamental measurement properties with less strict assumptions than parametric IRT models.
Keywords: double monotonicity model, DMM, item response theory, IRT, Mokken scaling, monotone homogeneity model, multilevel modeling, mokken package, nonparametric IRT, R, rater effects
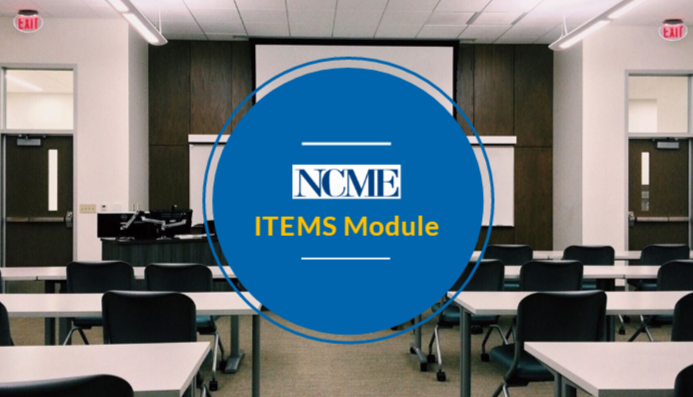
Digital Module 06: Posterior Predictive Model Checking
In this digital ITEMS module, Dr. Allison Ames and Aaron Myers discuss the most common Bayesian approach to model-data fit evaluation, which is called Posterior Predictive Model Checking (PPMC), for simple linear regression and item response theory models.
Keywords: Bayesian inference, simple linear regression, item response theory, IRT, model fit, posterior predictive model checking, PPMC, Bayes theorem, Yen’s Q3, item fit
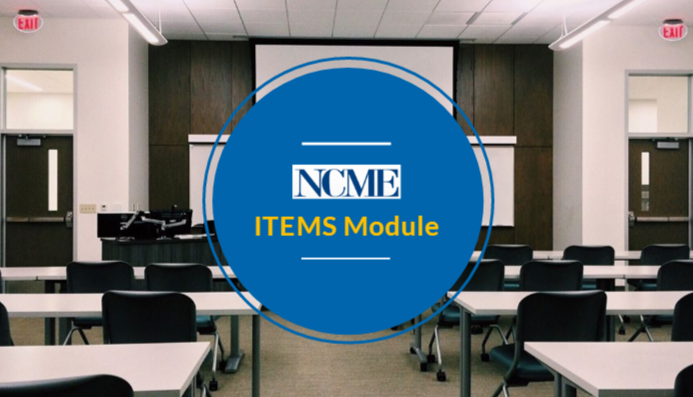
Digital Module 08: Foundations of Operational Item Analysis
In this digital ITEMS module, Dr. Hanwook Yoo and Dr. Ronald K. Hambleton provide an accessible overview of operational item analysis approaches for dichotomously scored items within the frameworks of classical test theory and item response theory.
Keywords: Classical test theory, CTT, corrections, difficulty, discrimination, distractors, item analysis, item response theory, operations, R Shiny, TAP, test development
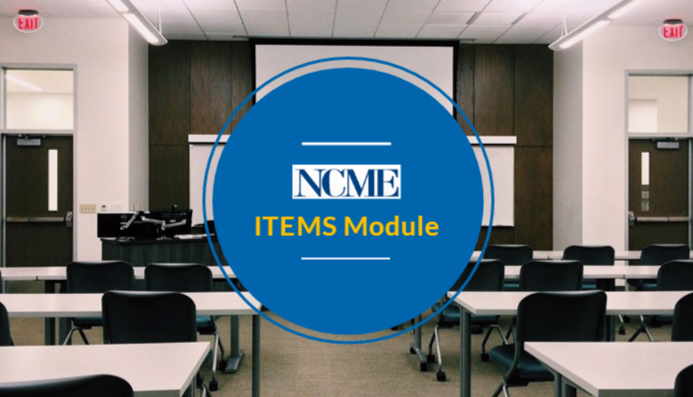
Digital Module 10: Rasch Measurement Theory
In this digital ITEMS module, Dr. Jue Wang and Dr. George Engelhard Jr. describe the Rasch measurement framework for the construction and evaluation of new measures and scales and demonstrate the estimation of core models with the Shiny_ERMA and Winsteps programs.
Keywords: invariance, item fit, item response theory, IRT, person fit, model fit, multi-faceted Rasch model, objective measurement, R, Rasch measurement, Shiny_ERMA, Winsteps
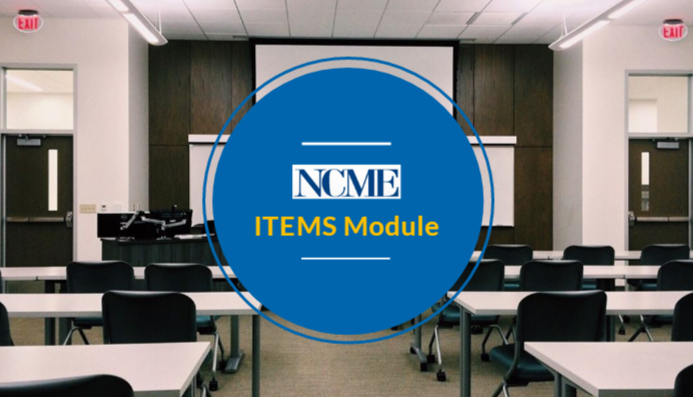
Digital Module 11: Bayesian Psychometrics
In this digital ITEMS module, Dr. Roy Levy discusses how Bayesian inference is a mechanism for reasoning in probability-modeling framework, describes how this plays out in a normal distribution model and unidimensional item response theory (IRT) models, and illustrates these steps using the JAGS software and R.
Keywords: Bayesian psychometrics, Bayes theorem, dichotomous data, item response theory (IRT), JAGS, Markov-chain Monte Carlo (MCMC) estimation, normal distribution, R, unidimensional models
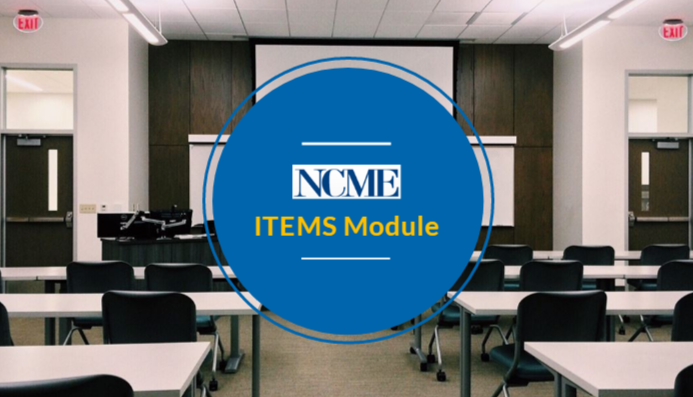
Digital Module 13: Simulation Studies in IRT
In this digital ITEMS module, Dr. Brian Leventhal and Dr. Allison Ames provide an overview of Monte Carlo simulation studies (MCSS) in item response theory (IRT). MCSS are utilized for a variety of reasons, one of the most compelling being that they can be used when analytic solutions are impractical or nonexistent because they allow researchers to specify and manipulate an array of parameter values and experimental conditions (e.g., sample size, test length, and test characteristics).
Key words: bias, bi-factor model, estimation, graded response model, item response theory, mean squared error, Monte Carlo, simulation, standard error, two-parameter logistic model
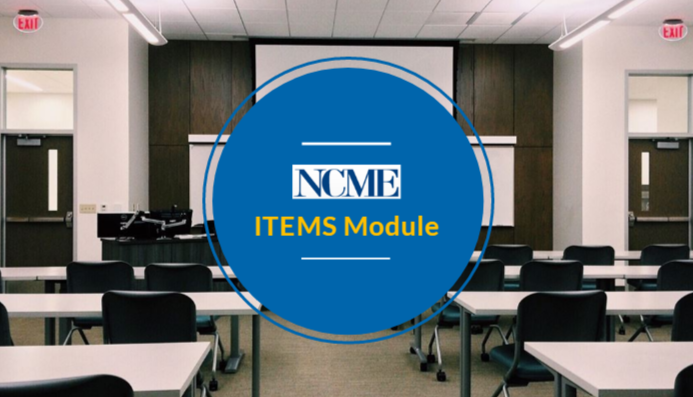
Digital Module 19: Foundations of IRT Estimation
In this digital ITEMS module, Dr. Zhuoran Wang and Dr. Nathan Thompson introduce the basic item response theory (IRT) item calibration and examinee scoring procedures as well as strategies to improve estimation accuracy.
Keywords: calibration, EM algorithm, estimation accuracy, item response theory (IRT), maximum likelihood estimation (MLE), maximum a posteriori (MAP), expected a posteriori (EAP), marginal maximum likelihood estimation (MMLE), scoring
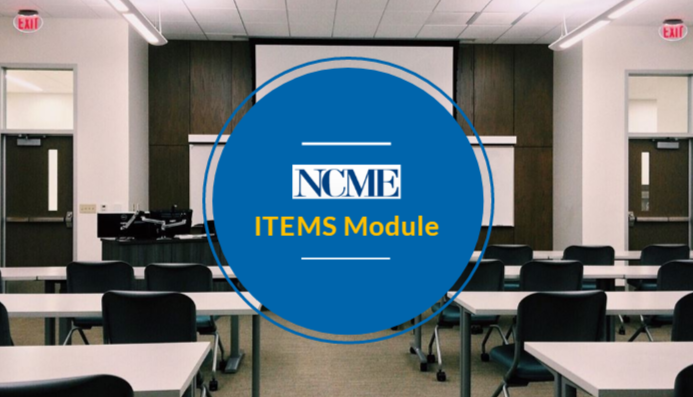
Digital Module 23: Multidimensional Item Response Theory Graphics
In this digital ITEMS module, Dr. Terry Ackerman and Dr. Qing Xie cover the underlying theory and application of multidimensional item response theory models from a visual perspective.
Keywords: centroid plot, clamshell plot, contour plot, item information curve, item information surface, multidimensional item response theory, MIRT, response surface, RShiny, test characteristic curve, test characteristic surface, vector
Access Date | Quiz Result | Score | Actions |
---|